Prompt Engineering Course in Hyderabad
- Master the art of giving prompts to Ai models like Chat GPT & Bard
- Online Live Training From Ai Experts in Prompt Engineering
- Recorded Videos of live sessions will be provided for lifetime
- Placement Assistance till you get the job
- Get Support in Resume Building and Interview Preparation
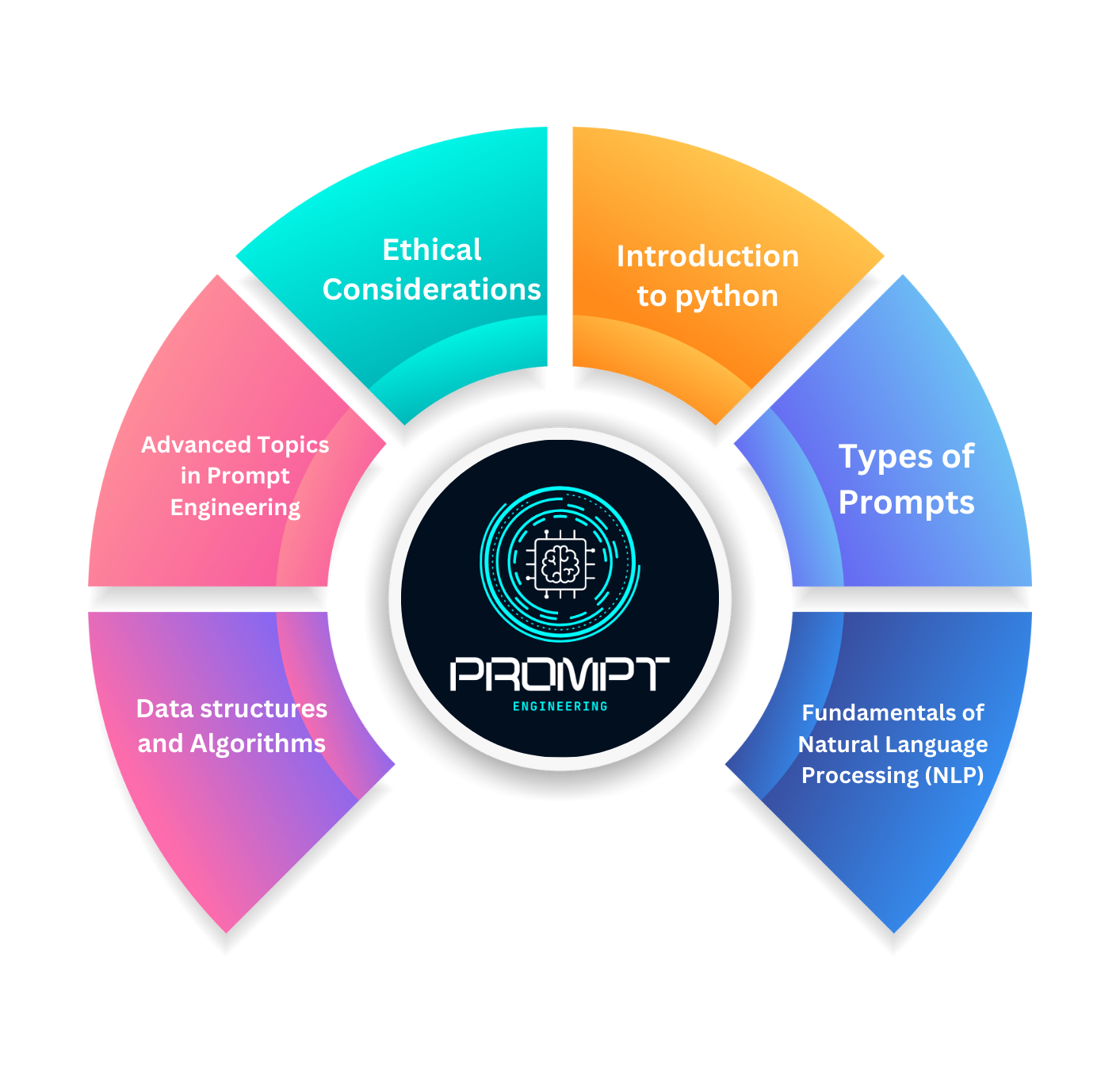
Table of Contents
TogglePrompt Engineering Course in Hyderabad
Batch Details
Trainers Name | Madhu, Dr.Prasad |
Trainers Experience | 12+ Years, 20+ Years |
Next Batch Date | 25th July 2024(offline) 12th August 2024(Online) |
Training Modes | Online and Offline Training (Instructor Led) |
Course Duration | 3 Months(Online&Offline) |
Call us at | +91 9052144555 |
Email Us at | brollyai.com@gmail.com |
Demo Class Details | Click here to chat on WhatsApp |
Prompt Engineering Course in Hyderabad
Course Topics
- Overview of Prompt Engineering
- Importance in AI and Natural Language Processing (NLP)
- Historical Context and Evolution
Basics of Python Programming
Installation and Setup:
● Installing Python and setting up a development environment (IDEs
like PyCharm, VSCode, Jupyter Notebooks)
Syntax and Basic Constructs:
● Variables and data types (integers, floats, strings, booleans)
● Basic input and output
● Comments and documentation
Control Structures
Conditional Statements:
● if, elif, else
Loops:
● for, while
● Loop control statements (break, continue, pass)
Functions
Defining Functions:
● Parameters and return values
Scope and Lifetime:
● Local and global variables
Lambda Functions:
● Anonymous functions
Core Data Structures
Lists:
● Creating, accessing, modifying, and iterating over lists
● List comprehensions
Tuples:
● Creating and using tuples
● Unpacking tuples
Sets:
● Creating and using sets
● Set operations (union, intersection, difference)
Dictionaries:
● Creating and using dictionaries
● Dictionary methods and comprehensions
Advanced Data Structures
Collections Module:
● defaultdict, Counter, OrderedDict, deque
File Operations
Reading and Writing Files:
● Opening, reading, writing, and closing files
● Working with different file modes (r, w, a, rb, wb)
Working with CSV and JSON:
● Reading from and writing to CSV and JSON files using csv and json
modules
OOP Basics
Classes and Objects:
● Defining classes and creating objects
● Instance variables and methods
Class Variables and Methods:
● Using class variables and class methods
Inheritance:
● Single and multiple inheritance
Polymorphism and Encapsulation:
● Method overriding
● Private variables and name mangling
Modular Programming
Creating and Importing Modules:
● Defining and using modules
Packages:
● Organizing code into packages
● Importing from packages
Error Handling
Exception Types:
● Common exceptions (ValueError, TypeError, etc.,)
Try, Except Blocks:
● Using try, except, else, and finally
Custom Exceptions:
● Creating and raising custom exceptions
Debugging
Debugging Techniques:
● Using print statements and logging
● Using debuggers (pdb)
Decorators and Context Managers
Decorators:
● Function decorators
● Class decorators
Context Managers:
● Using with statement
● Creating custom context managers with __enter__ and __exit__
Iterators and Generators
Iterators:
● Creating iterators using __iter__ and __next__
Generators:
● Creating generators with yield
● Generator expressions
NumPy:
● Arrays and matrix operations
Pandas:
● DataFrames for data manipulation
● Reading and writing data (CSV, Excel)
Data Visualization
Matplotlib:
● Plotting graphs and charts
Seaborn:
● Statistical data visualization
Unit Testing
Unittest Framework:
● Writing and running tests
● Test fixtures and test suites
Pytest:
● Advanced testing with pytest
Prompt Engineering Course in Hyderabad
Key Points
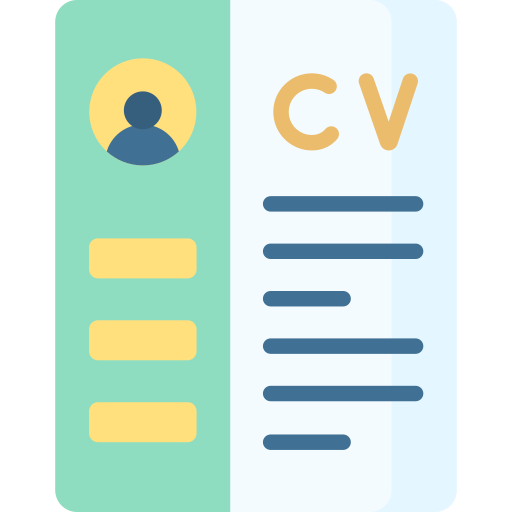
Comprehensive Curriculum
Brolly Ai provides a complete curriculum for Prompt Engineering training in Hyderabad, covering essential topics and practical skills required in the field.
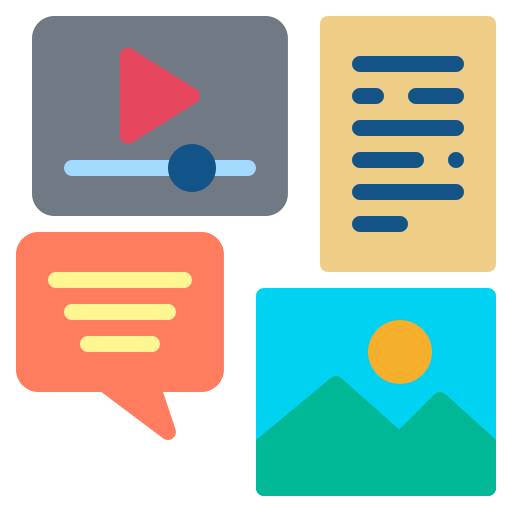
Industry-Relevant Content
The institute focuses on delivering content that is highly relevant to the industry, ensuring that students are well-prepared for real-time applications of Prompt Engineering.
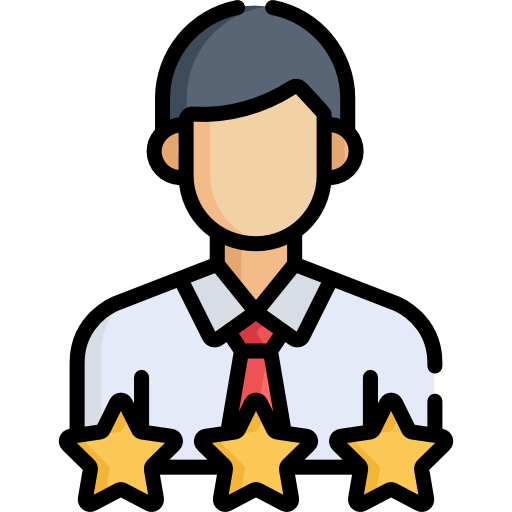
Experienced Instructors
The training is helped by experienced instructors who have both theoretical knowledge and practical experience in Prompt Engineering, Improving the overall learning experience.
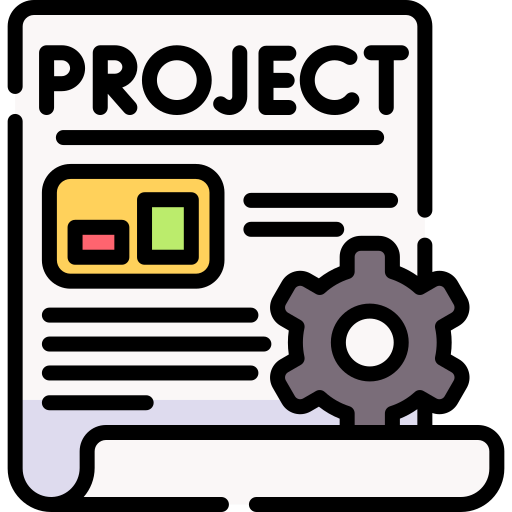
Hands-On Projects
Brolly Ai hands-on learning through projects, allowing students to apply their knowledge in practical scenarios and develop problem-solving skills.
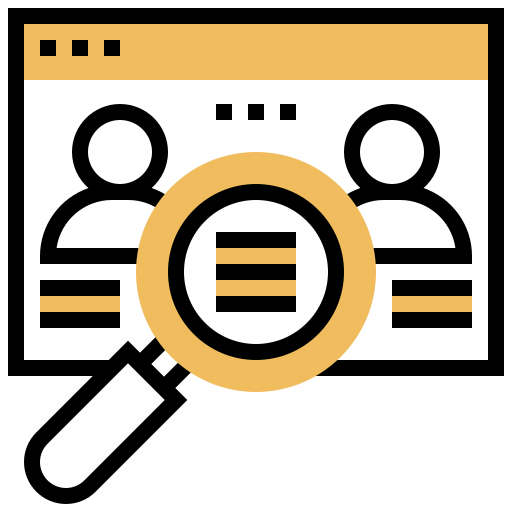
Placement Assistance
Brolly Ai offers placement assistance to its students, connecting them with potential employers and helping them secure job opportunities in the field of Prompt Engineering.
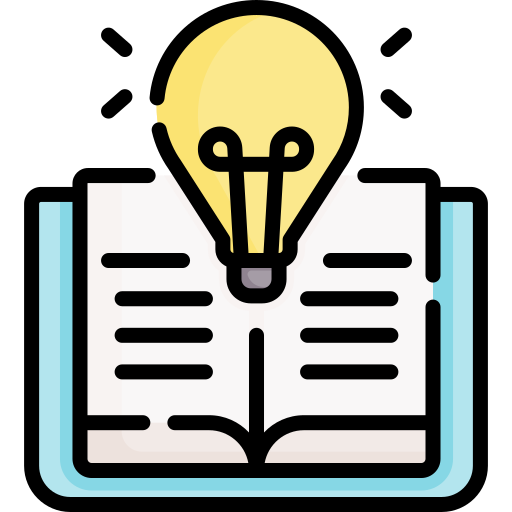
Flexible Learning Options
Recognizing the diverse needs of learners, the institute provides flexible learning options, including part-time courses, weekend classes, and online modules to accommodate varying schedules.
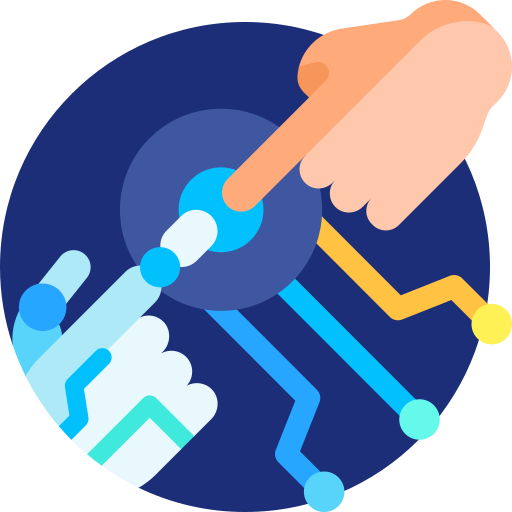
Networking Opportunities
Students at Brolly Ai have access to networking opportunities with industry professionals, developing connections that can be valuable for career growth and development.
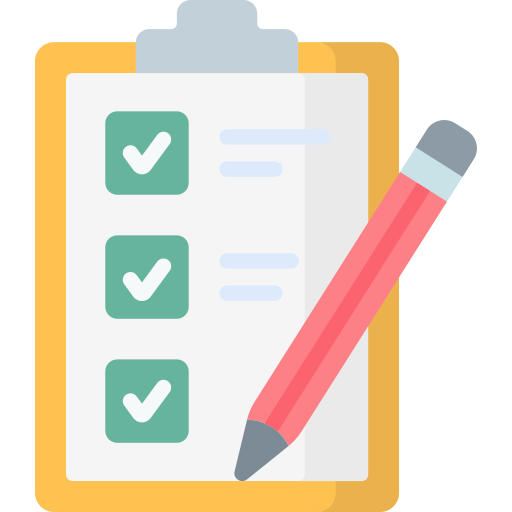
Continuous Learning Support
The institute provides continuous learning support through resources, workshops, and mentorship programs, ensuring that students stay updated with the latest advancements in Prompt Engineering even after completing their training.
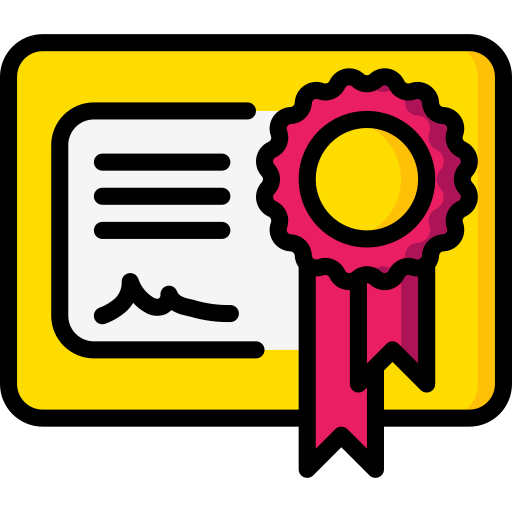
Certification Programs
Once you finish the Prompt Engineering training, the institute gives you course completion certificate. This certificate shows the employer's that you are excel in that particular field .This helps you find more job opportunities.
What is Prompt Engineering?
- Prompt Engineering is about creating clear instructions for artificial intelligence systems to generate specific and desired outputs.
- Prompt Engineers essentially work on improve the communication between humans and Ai systems, making interactions more effective and suitable to user needs.
- Prompt Engineering involves designing queries or requests that guide Ai models to provide accurate and relevant information or creative content.
- The goal of Prompt Engineering is to make AI models easier to understand and control and making them more user-friendly and aligned with specific tasks or goals.
- In Prompt Engineering, the focus is to create input prompts that help AI algorithms produce best possible results.This involves carefully designing the words and phrases you use when giving instructions to the AI.
- It plays a crucial role in adjusting language models, to make sure they produce desired responses in various applications like chatbots, content creation, and data analysis.
Prompt Engineering Course in Hyderabad
Pre-Requisites
- A fundamental understanding of programming languages, such as Python, is often needed for Prompt Engineering Training in Hyderabad.
- Having a basic knowledge of artificial intelligence concepts, including machine learning fundamentals, sets a solid foundation for individuals pursuing Prompt Engineering training.
- Familiarity with the basics of Natural Language Processing is beneficial, as Prompt Engineering often involves working with language models and text-based inputs.
- Proficiency in problem-solving, critical thinking, and logical reasoning, as these skills are crucial for effective prompt design and optimization.
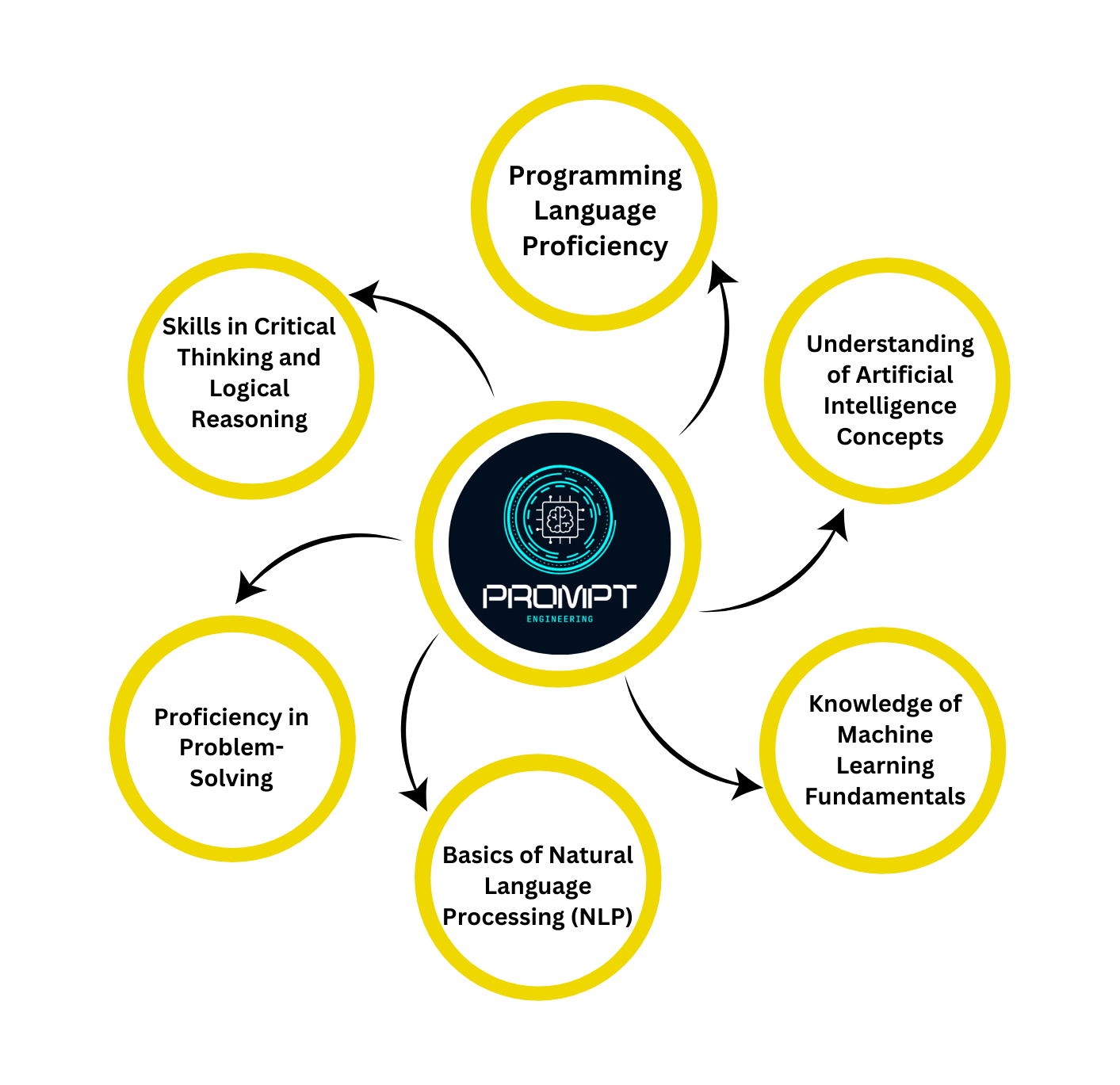
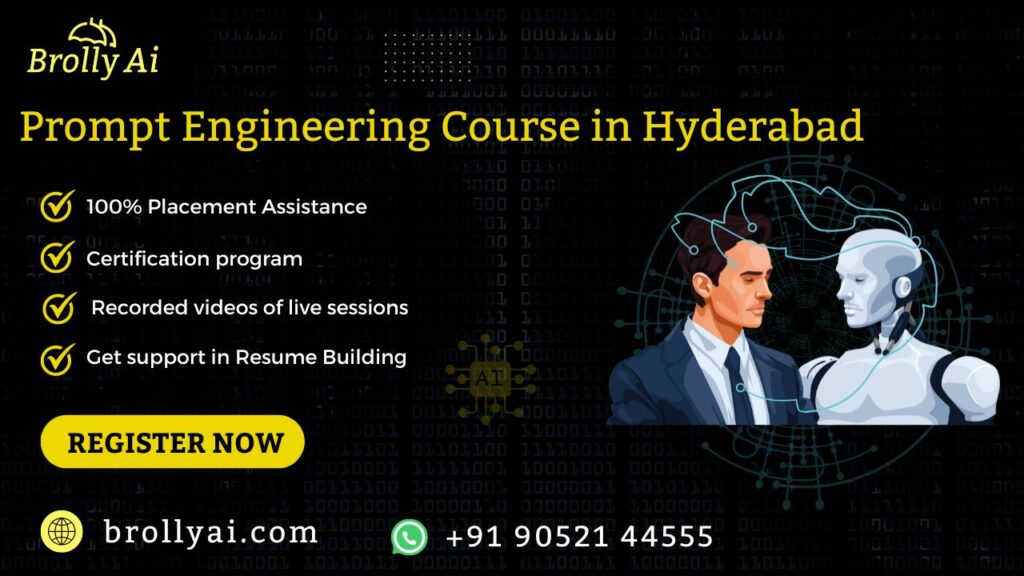
Prompt Engineering Course in hyderabad
Course Outline
01
The course begins with an introduction to Prompt Engineering, providing a foundational understanding of its importance and applications in artificial intelligence.
02
Students will learn into programming basics, with a focus on Python, to give them with the necessary skills for implementing prompt engineering techniques.
03
The course teaches important ideas in artificial intelligence, making sure participants understand machine learning basics and algorithms well.
04
The course includes a special module on Natural Language Processing (NLP), focusing on how language models and text processing are used in prompt design.
05
The course includes practical labs and simulations, letting students use what they learn in a real-time projects this will increase the students career opportunities.
06
The course then moves on to more advanced subjects, teaching techniques for making prompt inputs better and improving the performance of AI models.
07
In our prompt engineering course internships are offered to students this will benefit for them to use prompt engineering skills in real life situations.
08
The course concludes with a big project, where students to showcase their proficiency in prompt engineering and its applications in different AI situations.
Prompt Engineering Course in Hyderabad
Course Overview
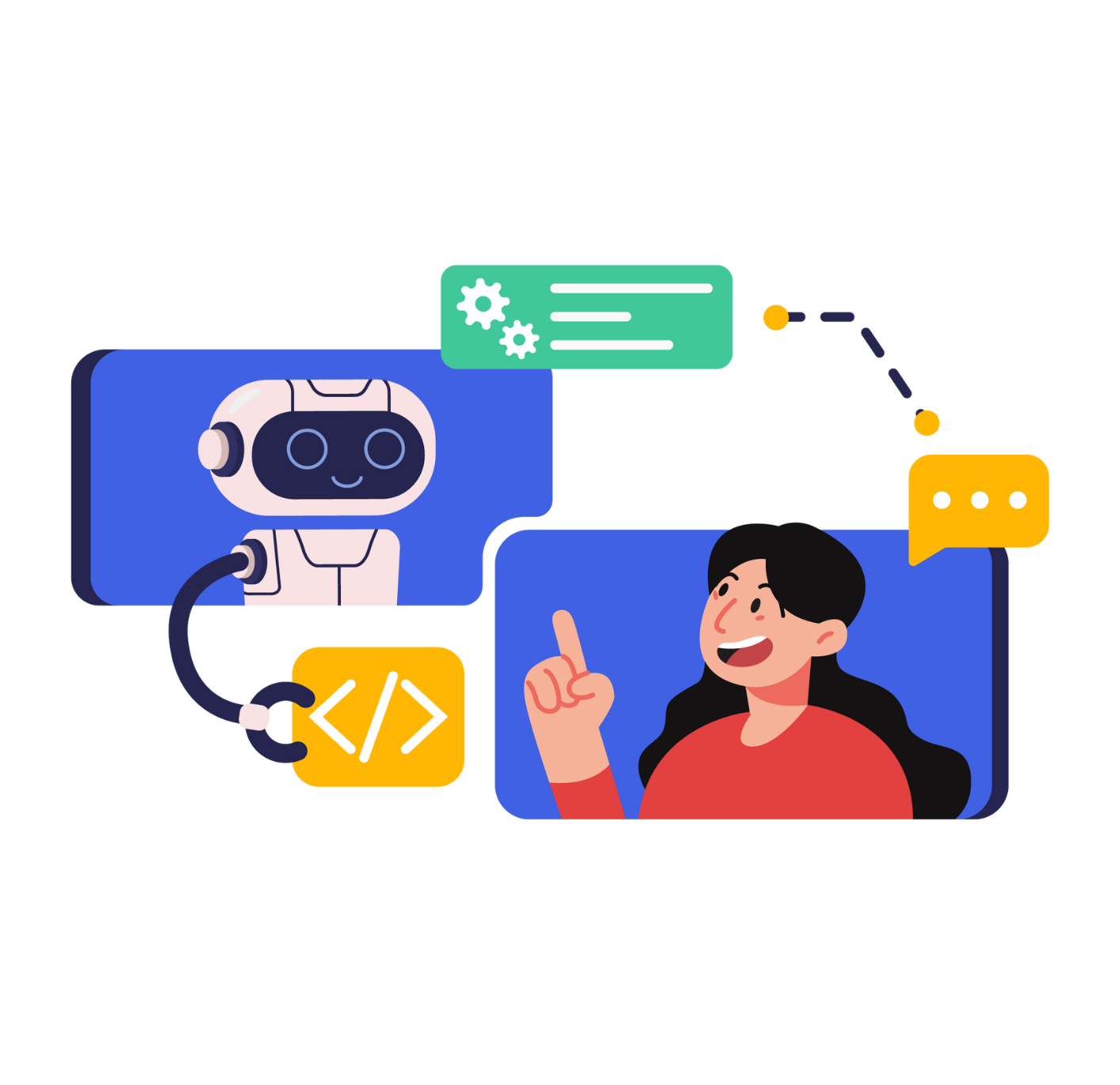
Brolly Ai‘s Prompt Engineering course in Hyderabad is designed to prepare learners with the essential skills needed to effectively communicate with and optimize artificial intelligence systems. The course begins with a detailed introduction to Prompt Engineering, highlighting its importance in influencing of Ai models. Students will learn into the fundamentals of programming, focusing on Python, to build a strong foundation for implementing prompt engineering techniques.
As the course progresses, participants will explore key concepts in artificial intelligence, with a specific focus on machine learning principles and algorithms. A dedicated module on Natural Language Processing (NLP) ensures that learners gain understanding into the role of language models and text processing in creating exact and effective prompts. Brolly Ai Institute’s Prompt Engineering course not only provides theoretical knowledge but also provides practical experience, preparing students for effective contributions in the dynamic field of Ai.
Modes Of Training
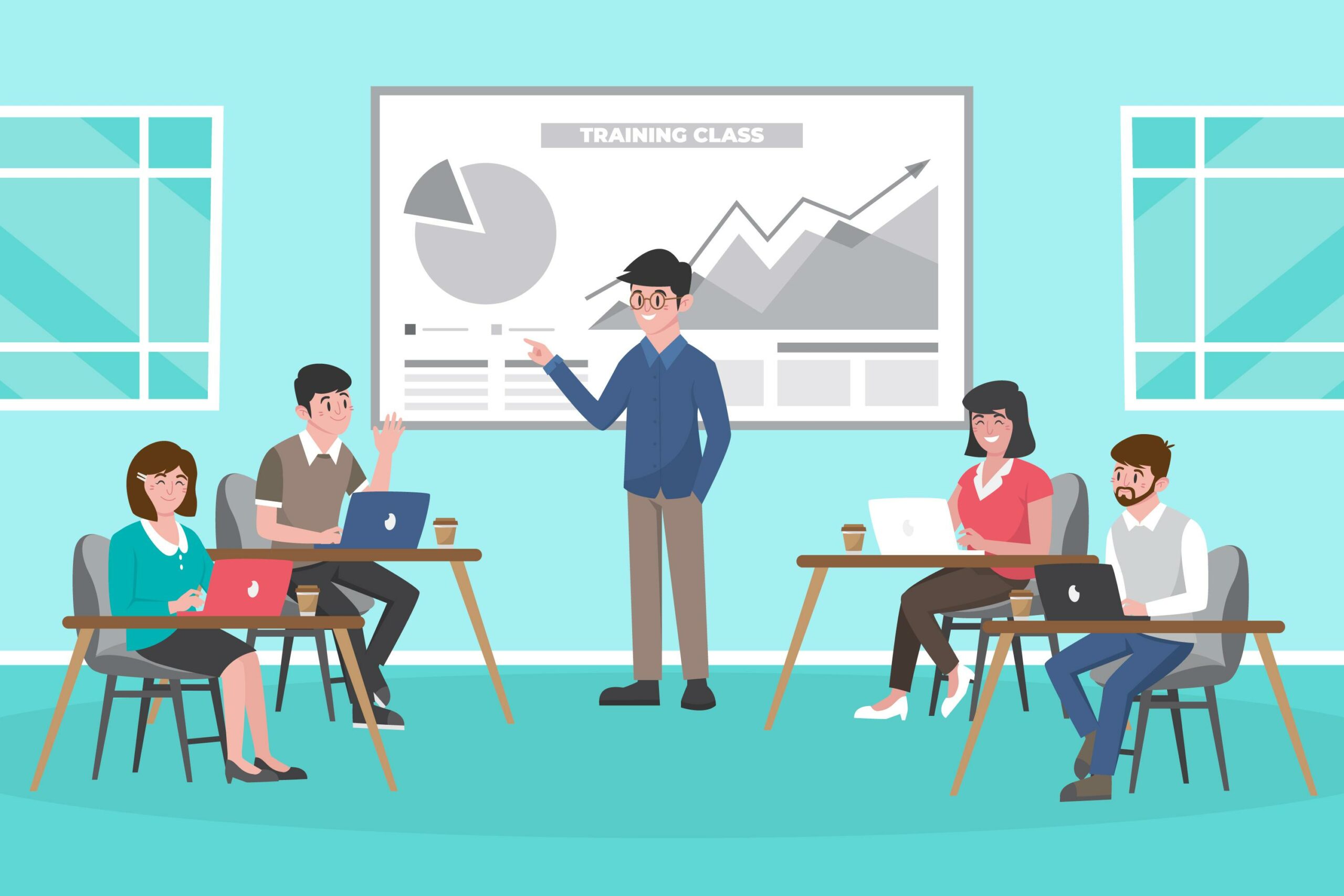
Classroom Training
- Interactive Classes
- Hands-on Workshops
- Group Discussions
- Instructor-Led Sessions
- On-site Learning
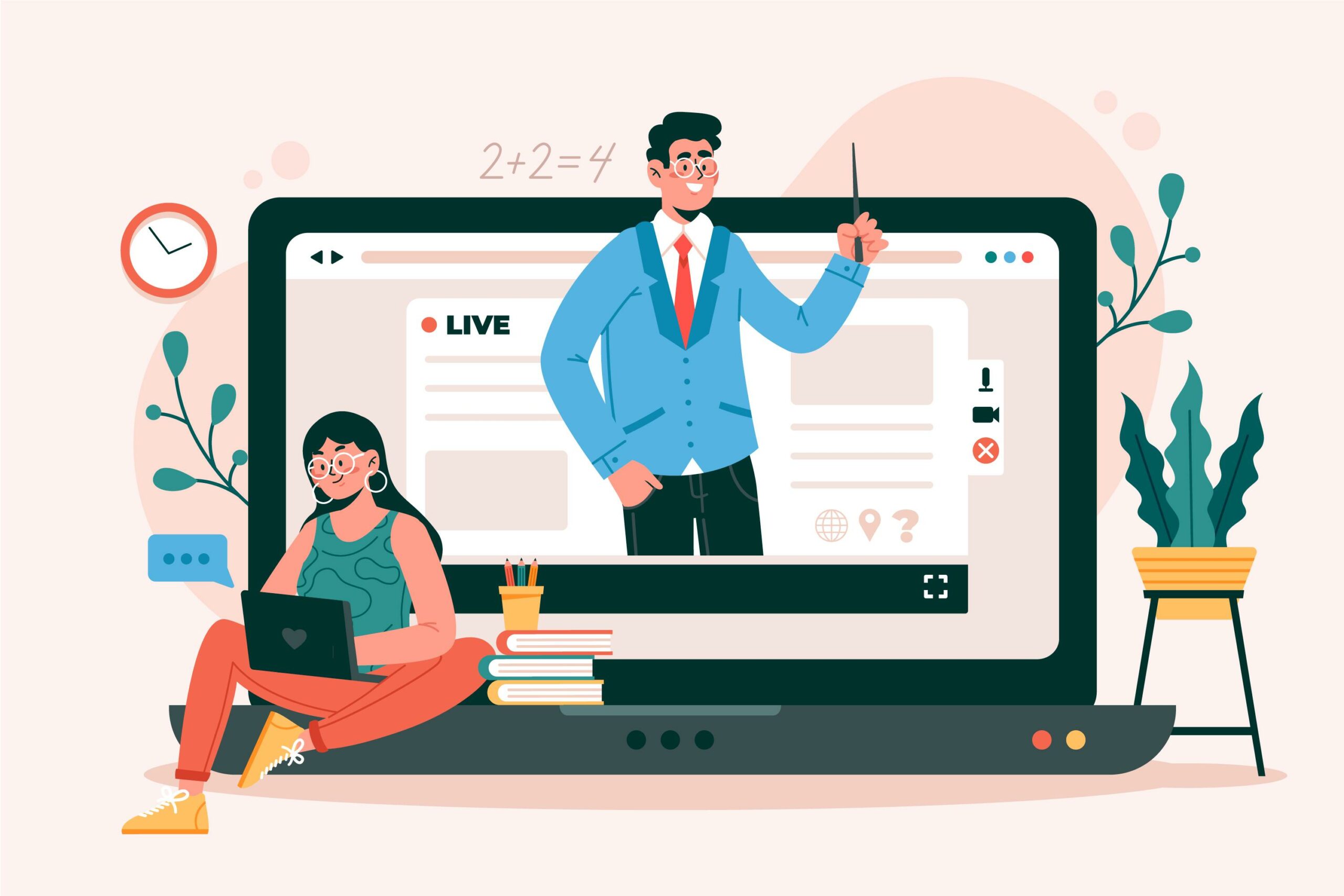
Online Training
- Online Classes
- Webinars
- E-Learning Modules
- Remote Labs
- Flexible Schedules
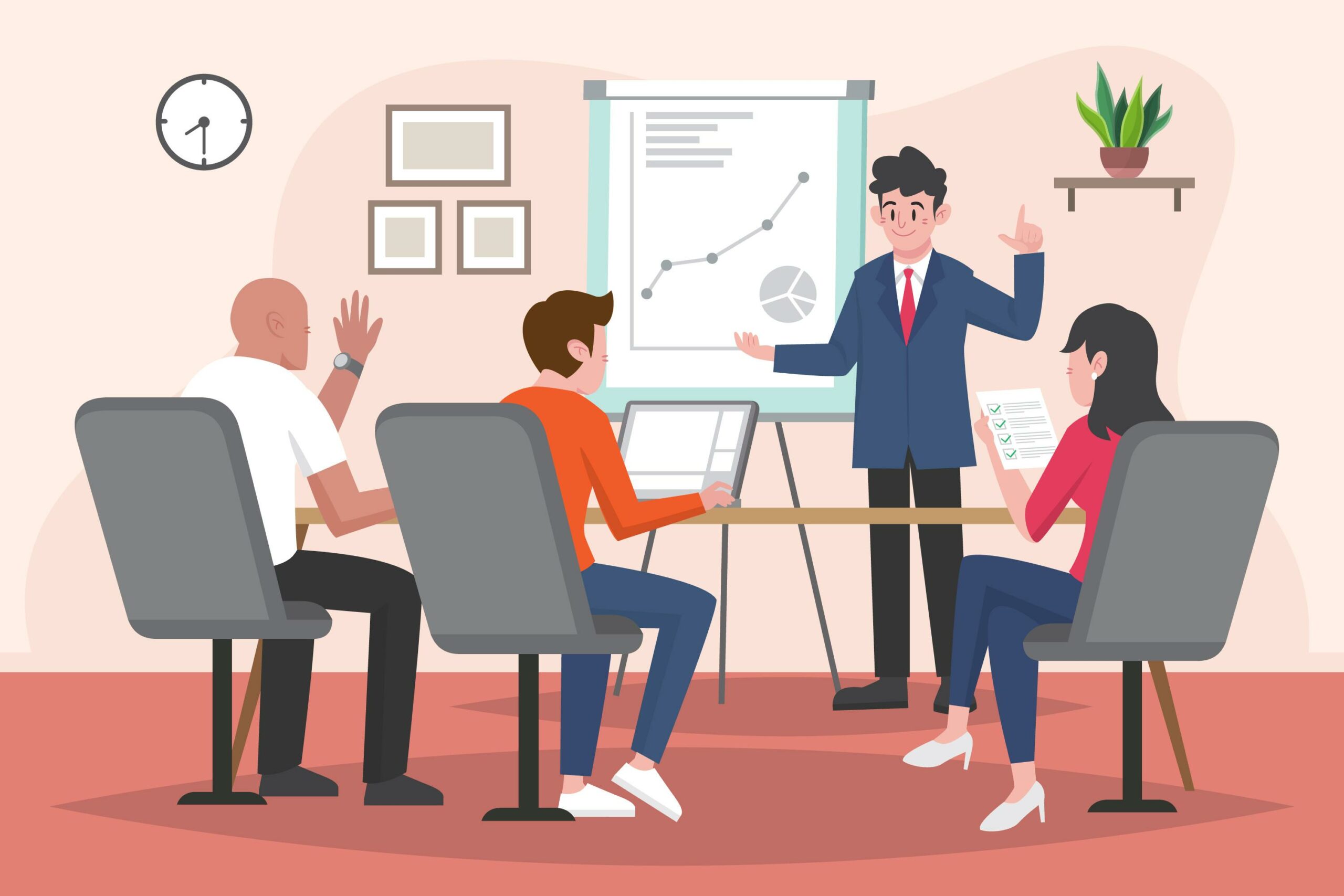
Corporate Training
- Personalized Programs
- Team Building
- Industry-Relevant Content
- On-Site Workshops
- Customized Learning Paths
Prompt Engineering Course in Hyderabad
Career Opportunities
01
Prompt Engineer: The primary role involves designing and improving prompts for Ai systems, ensuring they generate accurate and relevant outputs based on user queries.
02
AI Language Model Tuner: Responsible for optimizing language models by adjusting prompt inputs, optimizing model parameters, and enhancing overall performance.
03
Chatbot Developer: Specializing in creating prompts for chatbots, these professionals create conversational experiences by guiding the Ai in understanding and responding to user messages effectively.
04
Content Generation Specialist: Focused on using prompt engineering to generate creative and informative content, such as articles, summaries, or product descriptions, utilising Ai capabilities.
05
Data Analyst with Prompt Engineering Skills: Integrating prompt engineering techniques into data analysis tasks, these professionals optimize queries to acquire meaningful knowledge from large datasets.
06
Virtual Assistant Designer: Creating prompts for virtual assistants to improve user interactions , making the AI assistants more responsive and suited to users’ specific needs.
07
AI Product Manager: Managing the prompt design for AI products to meet user expectations and working with developers to improve how the products work.
08
User Experience (UX) Specialist with AI Focus: Using prompt engineering principles into the design of user platform to create AI-powered apps easy and smooth to use.
09
AI Researcher – Prompt Studies: Conducting research on prompt engineering methodologies, contributing to advancements in the field and developing best practices for effective prompt design.
10
Educator in AI and Prompt Engineering: Sharing knowledge and skills in prompt engineering through teaching and training, preparing the next generation of professionals in this developing field.
Prompt Engineering Course in Hyderabad
Certifications
The Prompt Engineering Certification offered by Brolly Ai is very Important in today’s rapidly changing tech environment. This certification not only validates an individual’s proficiency in creating accurate instructions for Ai systems but also indicates their ability to optimize and enhance the performance of language models. As the demand for Ai applications continues to grow, organizations seek professionals with specialized skills in prompt engineering to ensure effective communication between humans and Ai systems.
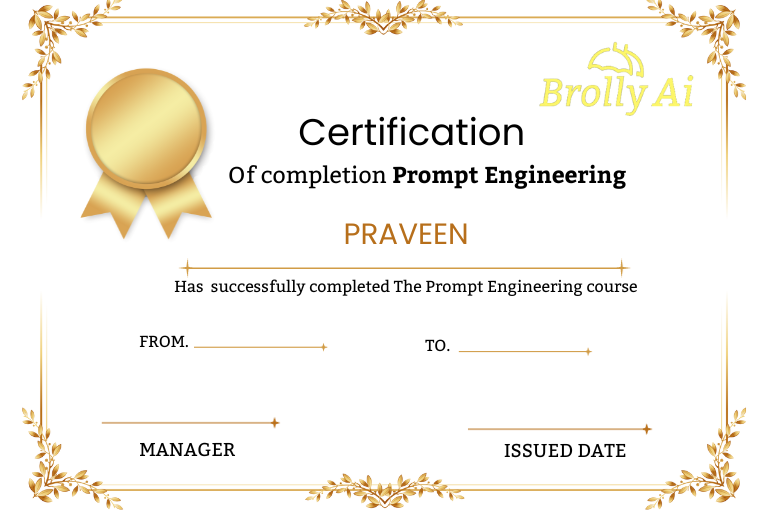
Testimonials
Sara Patel

@sarapatel
Raj Kumar

@raj kumar
Ananya Gupta

@ananyagupta
Amit Singh

@amitsingh
The Brolly Ai Prompt Engineering course is a career path . I went in with zero coding skills, and now I’m confidently creating prompts for Ai models. The hands-on projects were a game-changer. they also offer Generative Ai and Machine learning courses.Thank you Brolly AI for your wonderful support.
Priya Sharma

@priyasharma
Nikhil Verma

@nikhilverma
Prompt Engineering Course in Hyderabad
Benefits
Enhanced Communication with Ai
Prompt engineering training prepares individuals with the skills to effectively communicate with Ai systems, supporting them to create exact instructions and receive desired outcomes.
Career Advancement
Acquiring prompt engineering skills opens doors to various job roles in AI development, chatbot design, content creation, and more, providing more career opportunities in the expanding field of artificial intelligence.
Improved Problem-Solving
Training in prompt engineering develops problem-solving skills as individuals learn to optimize prompts, improving the efficiency and accuracy of Ai models in generating specific outputs.
Increased Employability
In a technology focused job market, prompt engineering certification improves employability, making individuals stand out to employers seeking professionals with expertise in adjusting Ai systems for optimal performance.
Versatility in AI Applications
Individuals with prompt engineering training gain adaptability in applying AI across different domains, from natural language processing to data analysis, making them valuable assets in various industries.
Personalized User Experiences
Learning prompt engineering allows professionals to create personalized user experiences in Ai applications, ensuring that human-Ai interactions are personalised to specific needs and preferences.
Prompt Engineering Course in Hyderabad
Market Trend
01
Companies are increasingly investing in prompt engineering to improve the accuracy and relevance of Ai-generated content.
02
The market trend shows a growing demand for professionals skilled in adjusting language models through effective prompt design.
03
As chatbots become more common, prompt engineering is gaining importance for creating conversational and user-friendly Ai interactions.
04
Prompt engineering is developing as a key factor in improving the clarity and control of complex Ai models.
05
Job postings for prompt engineering roles have seen a remarkable increase, indicating a rising demand for specialists in the field.
06
The trend reflects a shift towards more personalized Ai applications, motivating the need for experts who can optimize prompts for specific user contexts.
07
Organizations are recognizing the value of prompt engineering in content creation, data analysis, and other Ai-driven processes, inspiring its market growth.
08
Keeping up with prompt engineering techniques is important because AI technology keeps getting better. It helps us stay in adjust with the changes happening in artificial intelligence.
Frequently Asked Questions
FAQ’S
Prompt Engineering focuses on training individuals to effectively use language models like GPT-3 for various applications. Unlike traditional programming courses, it emphasizes generating human-like text based on prompts.
Brolly Ai is renowned for its expertise in artificial intelligence and offers a comprehensive curriculum, experienced instructors, and hands-on projects to ensure a robust learning experience.
Yes, the course is designed to accommodate learners of all backgrounds. Basic computer literacy is recommended, but no prior programming experience is necessary.
The duration of the course is for 3 months for Prompt Engineering offered by Brolly Ai.
The course includes hands-on projects that cover diverse applications such as chatbot development, content generation, and natural language understanding, allowing you to apply prompt engineering skills in real-world scenarios.
Yes, Brolly Ai Institute provides post-course support and may offer job placement assistance to help students transition into the workforce.
Yes, Brolly Ai provide online access to course materials and recorded lectures, ensuring flexibility for students to review content at their own pace
While no specific prerequisites are required, having a basic understanding of machine learning concepts and programming languages like Python can be beneficial.
Brolly Ai offers alumni resources, newsletters, or community forums where you can stay connected with fellow learners and stay informed about industry updates.
Students can explore diverse career paths, including roles in natural language processing, Ai research, chatbot development, content creation, and more. The skills gained are versatile and applicable across various industries.
Prompt Engineering Course in Hyderabad #1 Best Training Online
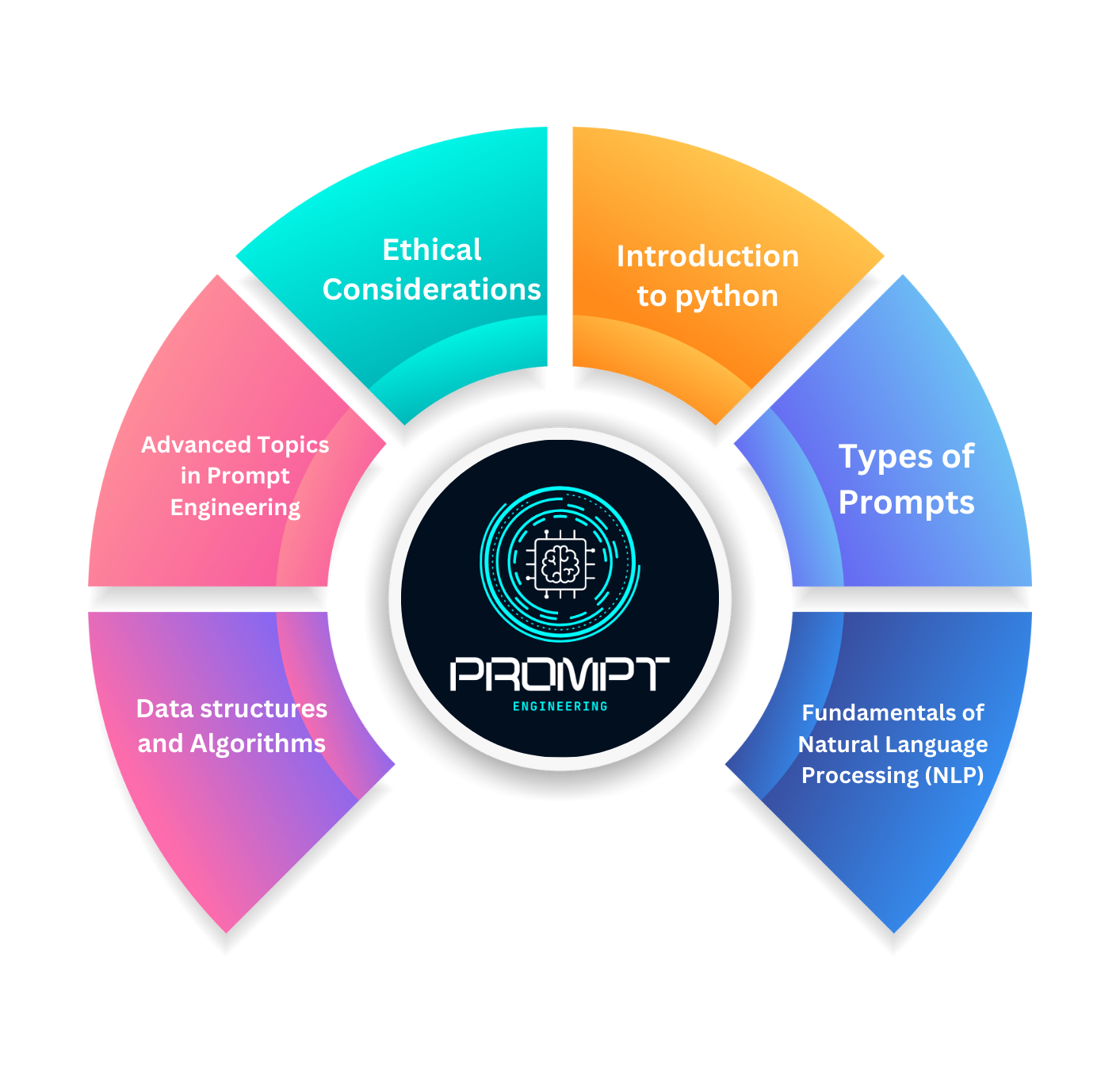
Brolly AI is the leading Training institute that offers prompt engineering course in Hyderabad with Placement Assistance. Best Online Training with Certification
Course Provider: Organization
Course Provider Name: Brolly AI
Course Provider URL: https://brollyai.com/
Course Mode: Onsite
Duration: 30:00:00
Repeat Count: 2
Repeat Frequency: Daily
Course Type: Paid
5